Data Science Innovations: Shaping the Future of Information
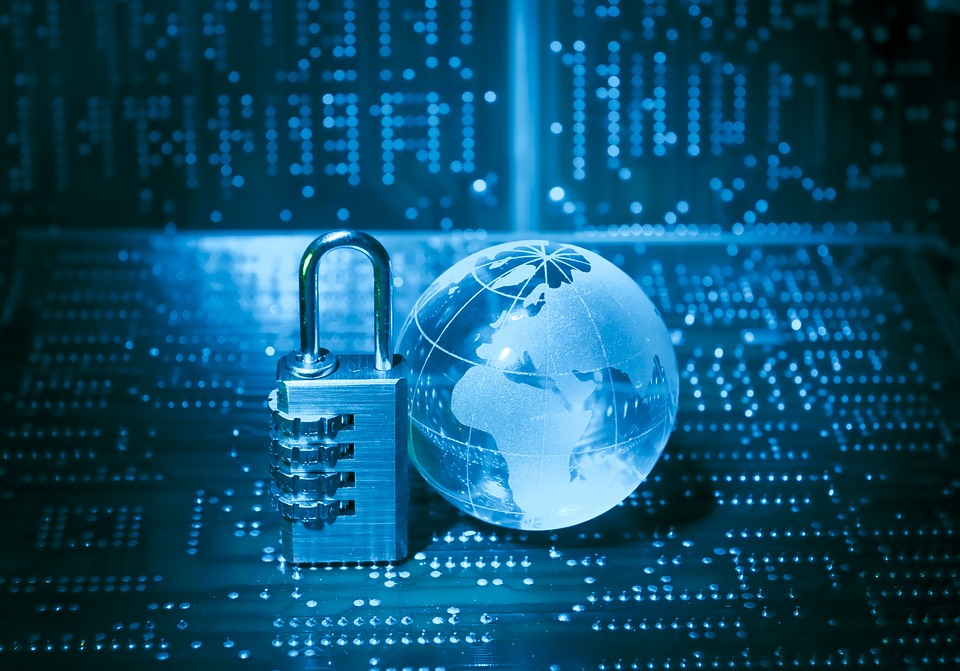
From influencing business strategies to reshaping industries, data science is at the forefront of technological innovation.
The Rise of Automated Machine Learning (AutoML)
One of the groundbreaking innovations in data science is Automated Machine Learning, or AutoML. This technology simplifies the process of applying machine learning models to complex data sets. Traditionally, building machine learning models required extensive expertise and time-consuming manual processes.
AutoML democratizes access to machine learning by automating model selection, feature engineering, and hyperparameter tuning.
AutoML tools have dramatically reduced the barrier to entry for businesses seeking to leverage machine learning. Companies can now deploy sophisticated predictive models without needing a team of data scientists, making advanced analytics accessible to small and medium-sized enterprises.
This innovation is not only accelerating the pace of machine learning adoption but also enabling organizations to derive insights from data more efficiently.
Explainable AI: Building Trust and Transparency
As artificial intelligence systems become more complex, the need for explainability has come to the forefront. Explainable AI (XAI) addresses this challenge by providing insights into how AI models make decisions. This transparency is crucial, particularly in sectors like healthcare and finance, where understanding the rationale behind a model’s prediction can have significant implications.
XAI techniques, such as SHAP (SHapley Additive exPlanations) and LIME (Local Interpretable Model-agnostic Explanations), help demystify the “black box” nature of AI models. By offering interpretable results, these tools foster trust among users and stakeholders, paving the way for broader acceptance and integration of AI technologies.
Real-Time Data Processing: The Edge Computing Revolution
The proliferation of IoT devices and the need for real-time data processing have spurred the rise of edge computing. This innovation allows data processing to occur closer to where data is generated, reducing latency and bandwidth usage. In industries like autonomous vehicles and smart cities, where real-time decision-making is critical, edge computing is a game-changer.
By decentralizing data processing, edge computing not only enhances efficiency but also addresses privacy concerns. Data can be processed locally, minimizing the need to transfer sensitive information to centralized cloud servers. This approach not only speeds up data processing but also ensures compliance with data protection regulations.
Data Science in Healthcare: Predictive Analytics for Better Outcomes
Healthcare is one of the sectors most profoundly impacted by data science innovations. Predictive analytics in healthcare leverages data to forecast patient outcomes, optimize treatment plans, and improve resource allocation. Machine learning models analyze data from electronic health records, medical imaging, and genomics to provide actionable insights.
For instance, predictive models can identify patients at risk of developing chronic conditions, enabling early intervention and personalized treatment plans. This proactive approach not only improves patient outcomes but also reduces healthcare costs by preventing complications and hospital readmissions.
The Role of Natural Language Processing in Data Science
Natural Language Processing (NLP) is another area where data science is making significant strides. NLP enables computers to understand and interpret human language, opening up a world of possibilities for data analysis. From sentiment analysis in social media to chatbots in customer service, NLP is transforming how businesses interact with their customers.
One of the most exciting applications of NLP is in the field of sentiment analysis. By analyzing customer feedback and social media posts, companies can gauge public sentiment towards their products and services. This real-time feedback loop allows businesses to make informed decisions and tailor their strategies to meet customer expectations.
Reinforcement Learning: A Breakthrough in AI Training
Reinforcement learning is a cutting-edge technique that is revolutionizing how AI models are trained. Unlike traditional supervised learning, reinforcement learning involves training models through trial and error, using feedback from their actions to improve performance.
This approach has proven particularly effective in environments where rules are not explicitly defined.
One of the most notable successes of reinforcement learning is in the realm of gaming. AI models trained using this technique have achieved superhuman performance in games like Go and chess, showcasing the potential of reinforcement learning to tackle complex problems.
Data Science Ethics: Navigating the Challenges of Innovation
As data science continues to evolve, ethical considerations are becoming increasingly important. The power of data science to influence decisions and shape societal outcomes necessitates a careful examination of ethical issues such as bias, privacy, and accountability.
Data scientists are now tasked with ensuring that their models are fair and unbiased. This involves scrutinizing data sources for potential biases and implementing strategies to mitigate their impact.
Additionally, privacy concerns must be addressed, particularly when dealing with sensitive data. Ethical data science requires a commitment to transparency and accountability, fostering trust among users and stakeholders.
Conclusion: Embracing the Future of Data Science
Data science innovations are undoubtedly transforming the way we live and work. From automated machine learning to the ethical challenges posed by AI, the field is constantly evolving. As we continue to harness the power of data, it is crucial to embrace these innovations while remaining mindful of their implications.
The future of data science holds immense potential, promising breakthroughs that will reshape industries and improve lives. By staying informed and engaged with these advancements, businesses and individuals alike can harness the opportunities presented by data science to drive growth and innovation.
As we look ahead, the challenge lies in balancing technological progress with ethical responsibility, ensuring that data science serves as a force for good in our ever-changing world.